Table Of Content
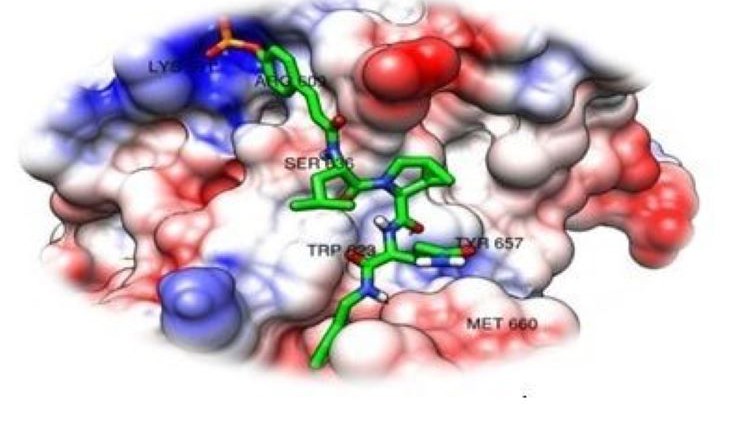
HDAC6 is a member of the class IIb Histone deacetylases (HDACs) family and is usually found in cytosol in association with non-histone proteins [77, 78]. The implementation of CADD has been reported to result in the design of a potential inhibitor of this enzyme. In one study conducted by Goracci et al., a virtual screening approach was used to identify potential inhibitors for HDAC6, and these were then subjected to in vitro testing.
"The Evolving Role of Modeling and Informatics in Drug Discovery"
The focused candidate ligand sets, predicted by such screening, often show useful (10–40%) hit rates in experimental testing60, yielding novel hits for many targets with potencies in the 0.1–10-μM range (for those that are published, at least). Further steps in optimization of the initial hits obtained from standard screening libraries of less than 10 million compounds, however, usually require expensive custom synthesis of analogues, which has been afforded only in a few published cases20,61. Identifying novel, potential drugs for NDs is difficult using traditional approaches of drug discovery [7]. However, during the last decade, computers have been used to aid and accelerate the process of drug discovery, and this process is now referred to as computer-aided drug design (CADD) or computer-assisted molecular design (CAMD). Neurodegenerative disorders (NDs) are diverse group of disorders characterized by escalating loss of neurons (structural and functional). The development of potential therapeutics for NDs presents an important challenge, as traditional treatments are inefficient and usually are unable to stop or retard the process of neurodegeneration.
Data Availability
LBDD is generally categorized as Quantitative Structure Activity Relationship (QSAR) or pharmacophore modeling. In this review, we have briefly described about CADD and its use in the development of the therapeutic drug candidates against NDs. The successful applications, limitations and future prospects of this approach have also been discussed. Feature papers represent the most advanced research with significant potential for high impact in the field. A FeaturePaper should be a substantial original Article that involves several techniques or approaches, provides an outlook forfuture research directions and describes possible research applications. Dr. Pellecchia is a Professor of Biomedical Sciences at the School of Medicine of the University of California Riverside (UCR) and is the founding Director of the Center for Molecular and Translational Medicine at UCR.
Optibrium Acquires BioPharmics LLC, Expanding Its 3D Drug Design and Visualisation Offering - Technology Networks
Optibrium Acquires BioPharmics LLC, Expanding Its 3D Drug Design and Visualisation Offering.
Posted: Wed, 06 Sep 2023 07:00:00 GMT [source]
Meet the UC Campus Leads
This includes structure-based virtual screening of gigascale chemical spaces, further facilitated by fast iterative screening approaches. Highly synergistic are developments in deep learning predictions of ligand properties and target activities in lieu of receptor structure. Here we review recent advances in ligand discovery technologies, their potential for reshaping the whole process of drug discovery and development, as well as the challenges they encounter. We also discuss how the rapid identification of highly diverse, potent, target-selective and drug-like ligands to protein targets can democratize the drug discovery process, presenting new opportunities for the cost-effective development of safer and more effective small-molecule treatments. The long-used traditional methodology for novel drug discovery and drug development is an immensely challenging, multifaceted, and prolonged process.
Moreover, thousands of easily synthesizable analogues assure extensive SAR-by-catalogue for the best hits, which, for example, enabled approximately 100-fold potency and selectivity improvement for the CB2 V-SYNTHES hits26. Availability of the multilayer on-demand chemical space extensions (for example, supported by MADE building blocks47) can also greatly streamline the next steps in lead optimization through ‘virtual MedChem’, thus reducing extensive custom synthesis. The limited size and diversity of screening libraries have long been a bottleneck for detection of novel potent ligands and for the whole process of drug discovery. An average ‘affordable’ high-throughput screening (HTS) campaign29 uses screening libraries of about 50,000–500,000 compounds and is expected to yield only a few true hits after secondary validation. Those hits, if any, are usually rather weak, non-selective, have suboptimal ADMET and PK properties and unknown binding mode, so their discovery entails years of painstaking trial-and-error optimization efforts to produce a lead molecule with satisfying potency and all the other requirements for preclinical development. Scaling of HTS to a few million compounds can be afforded only in big pharma, and it still does not make that much difference in terms of the quality of resulting hits.
2. Quantitative Structure-Activity Relationships (QSARs)
His research laboratory focuses on the design of novel pharmacological tools and therapeutics in oncology, neurodegeneration, and other disease areas. Dr. Rogawski is Professor of Neurology and Pharmacology at the University of California, Davis School of Medicine. His research encompasses discovery of neurological therapeutics, characterization of drug mechanism, and early and later stage drug development. Dr. Rogawski is an elected fellow of the American Association for the Advancement of Science and was awarded the UC Davis Chancellor's Innovator of the Year Award for inventing the drug Zulresso™.
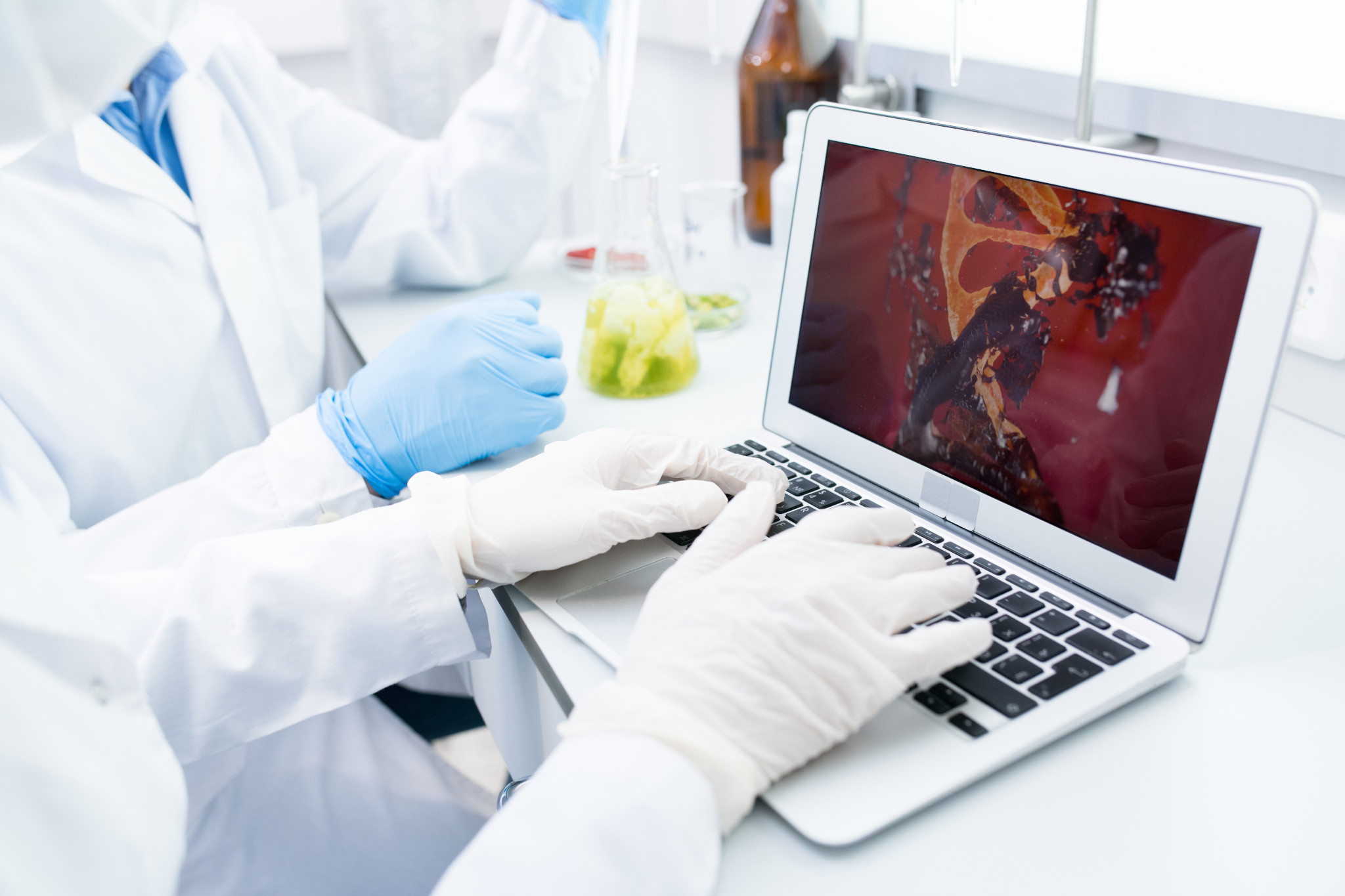
Artificial Intelligence For Drug Development and Discovery Market 2022
Moreover, the high failure rate in clinical trials (currently 90%)2 is largely explained by issues rooted in early discovery such as inadequate target validation or suboptimal ligand properties. Although the SBDD approach has proved as a pioneer in drug designing, it has to cross the challenges that the community has to look upon. This enhancement includes screening methods, chemogenomic compounds, data improvement, quantity, quality of various tools and databases, modifying in multitarget drug structures, toxicity predicting algorithms, integrating the approach for better efficacy and compatibility. The other most sounding parameter is electrostatic interactions, entropy calculations were ignored completely. Above all, there is no single software or package that works well for particular targets and ligands including the water molecule, and probable confirmation for the target and other innovations are still need to be addressed.
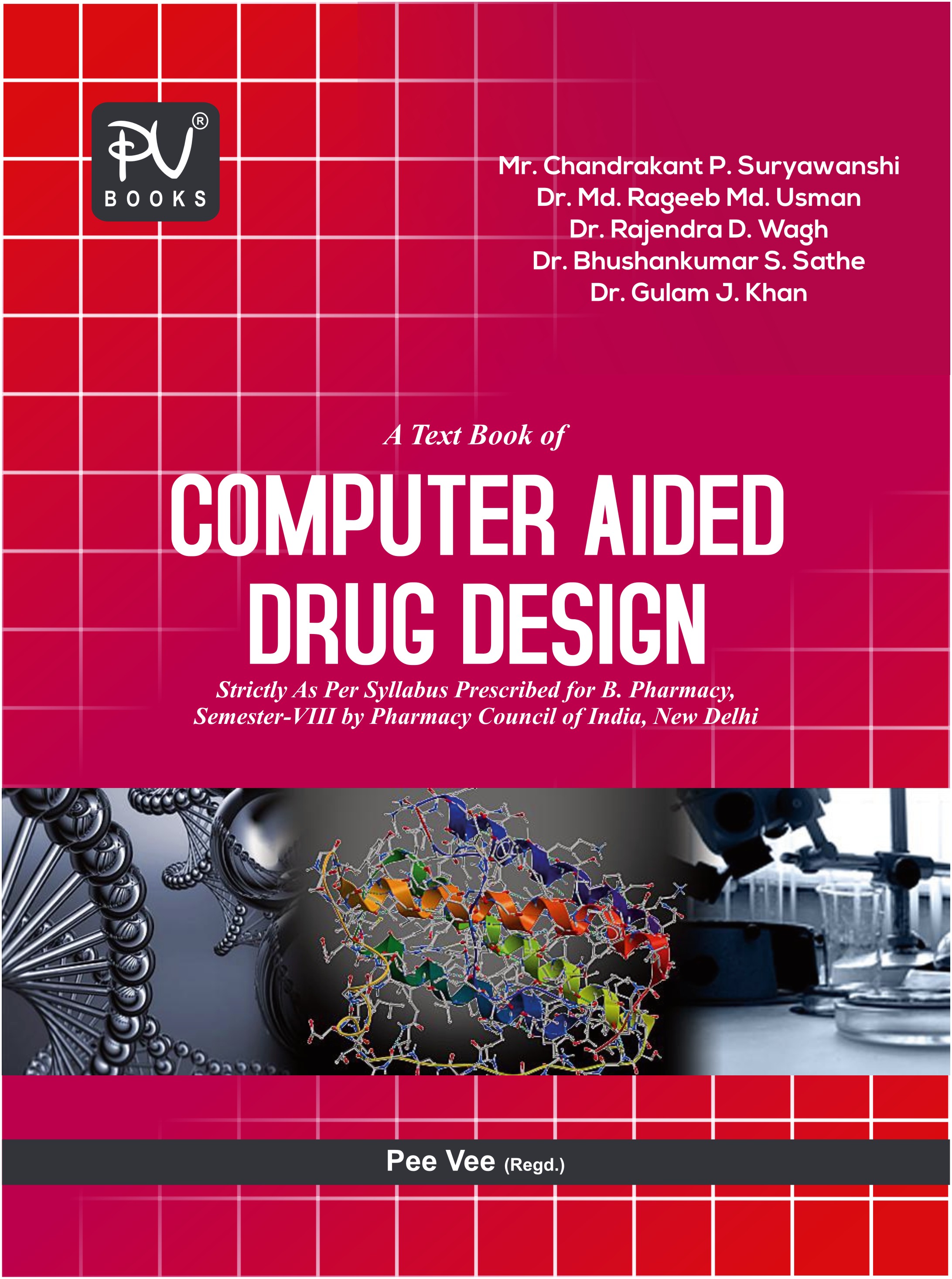
Molecular Docking and Structure-Based Drug Design
The recent examples of hybrid fragment-based computational design approaches targeting SARS-CoV-2 inhibitors highlight the challenges presented by such targets and allow head-to-head comparisons to ultra-large VLS. One of the studies was aimed at the SARS-CoV-2 NSP3 conserved macrodomain enzyme (Mac1), which is a target critical for the pathogenesis and lethality of the virus. Building on crystallographic detection of the low-affinity (180 μM) fragments weakly binding Mac1 (ref. 139), merging of the fragments identified a 1-μM hit, quickly optimized by catalogue synthesis to a 0.4-μM lead140. In the same study, an ultra-scale screening of 400 million REAL database identified more than 100 new diverse chemotypes of drug-like ligands, with follow-up SAR-by-catalogue optimization yielding a 1.7-μM lead140. For the SARS-CoV-2 main protease Mpro, the COVID Moonshot initiative published results of crystallographic screening of 1,500 small fragments with 71 hits bound in different subpockets of the shallow active site, albeit none of them showing in vitro inhibition of protease even at 100 μM (ref. 141). Numerous groups crowdsourcing the follow-up computational design and screening of merged and growing fragments helped to discover several SAR series, including a non-covalent Mpro inhibitor with an enzymatic IC50 of 21 μM.
The best models achieved a Spearman rank coefficient of 0.53 with a root-mean-square error of 0.95 for the predicted versus experimental pKd values in the challenge set. Such accuracy was found to be on par with the accuracy and recall of single-point experimental assays for kinase inhibition, and may be useful in screenings for the initial hits for less explored kinases and guiding lead optimization. Note, however, that the kinase family is unique as it is the largest class of more than 500 targets, all possessing similar orthosteric binding pockets and sharing high cross-selectivity. The distant second family with systematic cross-reactivity comprises about 50 aminergic GPCRs, whereas other GPCR families and other cross-reactive protein families are much smaller.
In addition to the common methods introduced in the first edition of this chapter, additional CADD methods developed recently in our laboratory as well as from other laboratories will be described below. The CADD Users Manual establishes the CADD standards using US Customary Units (English) and covers many of the resource files needed to complete a project within the Caltrans' right of way. This manual is subject to changes that reflect Caltrans' current development/delivery process using MicroStation and Civil3D for all projects on the State Highway System (SHS). The UC DDC was created by the University of California Biomedical Research Acceleration, Integration & Development (UC BRAID) Drugs, Devices, Diagnostics, Development (D4) group, and it has since expanded to actively include industry sponsors. Support for the consortium has been provided by a UC Office of the President (UCOP) Multi-Campus Research Proposal Initiative grant (MRPI) and its industry sponsor(s).
Such identified non-β-lactam-based β-lactamase inhibitors have the potential to be used in combination therapy with lactam-based antibiotics against multi-drug resistant clinical isolates. A combination of advanced computational techniques, biological science, and chemical synthesis was introduced to facilitate the discovery process, and this combinational approach enhanced the scale of discovery. Eventually, the term computer-aided drug design (CADD) was adopted for the use of computers in drug discovery [17, 18]. Advanced computational applications have been shown to be effective tools and notable successes have been achieved using these techniques. CADD is a specialized discipline, whereby different computational methods are used to simulate interactions between receptors and drugs in order to determine binding affinities [19]. However, the technique is not limited to studies of chemical interactions and binding affinity predictions, as it has many more applications ranging from the design of compounds with desired physiochemical properties to the management of digital repositories of compounds.
No comments:
Post a Comment