Table Of Content
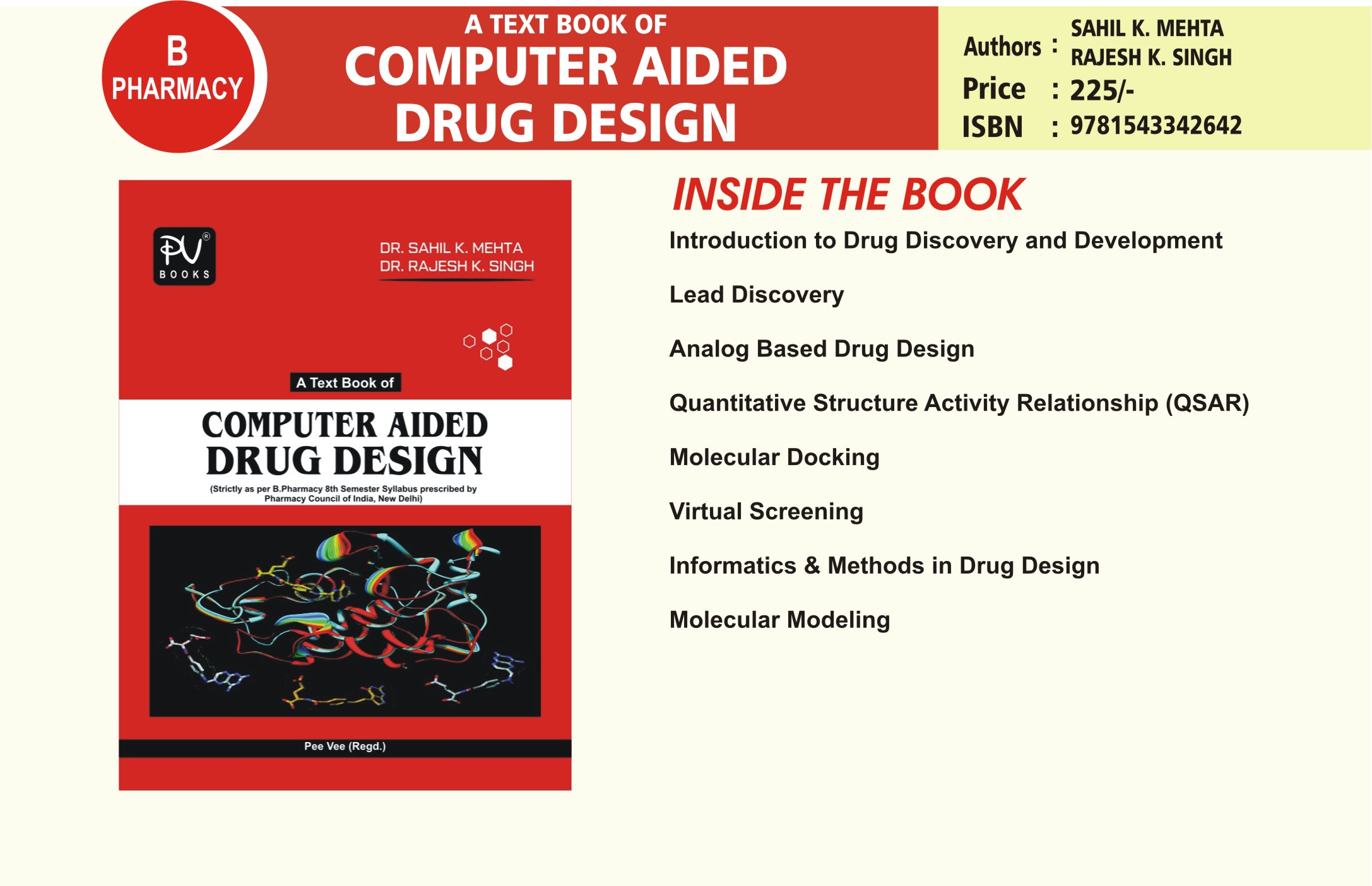
Further growth of the on-demand chemical space size and diversity is also supported by recent development of new robust reactions for the click-like assembly of building blocks. As well as ‘classical’ azide-alkyne cycloaddition click chemistry120, recognized by the 2022 Nobel Prize in chemistry121, and optimized click-like reactions including SuFEx122, more recent developments such as Ni-electrocatalysed doubly decarboxylative cross-coupling123 show promise. Other carbon–carbon forming reactions use methyliminodiacetic acid boronates for Csp2–Csp2 couplings124, and most recently tetramethyl N-methyliminodiacetic acid boronates125 for stereospecific Csp3–C bond formation. Each of these reactions applied iteratively can generate new on-demand chemical spaces of billions of diverse compounds operating with a limited number of building blocks.
Computer Aided Drug Design and its Application to the Development of Potential Drugs for Neurodegenerative Disorders
The quality of QSAR models, however, differs for different target classes depending on data availability, with the most advances achieved for the kinase superfamily and aminergic GPCRs. An unbiased benchmark of the best ML QSAR models was given by a recent IDG-DREAM Drug-Kinase Binding Prediction Challenge with the participation of more than 200 experts78. The top predictive models in this blind assessment included kernel learning, gradient boosting and DL-based algorithms. The top-performing model (from team Q.E.D) used a kernel regression, protein sequence similarity and affinity values of more than 60,000 compound–kinase pairs between 13,608 compounds and 527 kinases from ChEMBL79 and Drug Target Commons80 databases as the training data. The best DL model used as many as 900,000 experimental ligand-binding data points for training, but still trailed the much simpler kernel model in performance.
Machine learning accelerates pharmacophore-based virtual screening of MAO inhibitors
In collaborative studies with the Wilks lab, we have successfully applied CADD techniques to identify inhibitors of the bacterial heme oxygenases from Pseudomonas aeruginosa and Neisseria meningitides, thereby confirming the potential role of heme oxygenases as a novel antimicrobial targets (13, 14). A pharmacophore is an assembly (3D arrangement) of 'steric' and 'electronic' features required for optimal supramolecular interaction with a specific biological target structure and to prompt/block its biological response [63]. Ligand-based pharmacophore model generation is based on available information on the biological activities of compounds/ligands. A pharmacophore does not symbolize an actual molecule/ligand or real connection between functional groups, but rather provides an abstract description of molecular features that are vital for molecular interactions between molecules and macromolecular ligands. 3Protonation states of titratable residues at the targeted binding site and in the ligand being studied are quite important when setting up the CADD calculations.
Meet the UC Campus Leads
Upon target validation process, identification of hits and lead discovery phases has to be developed for a novel drug discovery process. Although, physical and biochemical parameters were used to decide the change in the structural property of the compounds to synthesize an effective lead molecule for the development of the drug. Several natural leads have become available in various databases and the literature with biological activity against its specific target, which leads to chemical modification. The most efficient and powerful process which governs entire drug discovery is structure-based drug designing (SBDD). The information about the target of small molecules, genetic information with their sequences, binding information, cytotoxicity, absorption, metabolism, excretion(ADMET) data, and other important biological information serve as the most efficient sources for accelerating the drug discovery process.
The development of more robust generative chemical spaces can also be supported by new computational approaches in synthetic chemistry, for example, predictions of new iterative reaction sequences129 or synthetic routes and feasibility from DL-based retrosynthetic analysis130. In generative models, synthesizability predictions can be coupled with predictions of potency and other properties towards higher levels of automated chemical design131. Thus, generative adversarial networks combined with reinforcement learning (GAN-RL) were recently used to predict synthetic feasibility, novelty and biological activity of compounds, enabling the iterative cycle of in silico optimization, synthesis and testing of the ligands in vitro50,132. When applied within a set of well-established reactions and pharmacologically explored classes of targets, these approaches already yield useful hits and leads, leading to clinical candidates50,132. However, the wider potential of automated chemical design concepts and robotic synthesis in drug discovery remains to be seen.
Sr Principal Research Scientist / Associate Director, Medicinal Chemistry
A number of tools and software have been developed for pharmacophore development, such as, Phase, Catalyst/Discovery Studio, MOE, and LigandScout [64]. LBDD offers a general approach for elucidating relationships between the structural and physicochemical properties of compounds/ligands and their biological activities. In this process the available information of ligands and their biological activity is used for the development of new potential drug candidates. LBDD is widely used in pharmaceutical research, as more than 50% of approved drugs targeting membrane proteins (for which 3D structures are often not available, such as, GPCR).

In its most common form, it involves modification of a known active scaffold or linking known active scaffolds, although de novo drug design (i.e., from scratch) is also possible. Though highly interrelated, identification of active scaffolds should be conceptually separated from drug design. Traditionally, the drug design process has focused on the molecular determinants of the interactions between the drug and its known or intended molecular target. Most antibiotics were designed to target proteins involved in intracellular processes, thus the outer membrane of bacteria needs to be penetrated for antibiotics to function.

At the same time, experimental testing of predictions also provides data that can feed back into improving the quality of the models by expanding their training datasets, especially for the ligand property predictions. Thus, the DL-based QSPR models will greatly benefit from further accumulating data in cell-permeability assays such as CACO-2 and MDCK, as well as new advanced technologies such as organs-on-a-chip or functional organoids to provide better estimates of ADMET and PK properties without cumbersome in vivo experiments. The ability to train ADMET and PK models with in vitro assay data representing the most relevant species for drug development (typically mouse, rat and human) would also help to address species variability as a major challenge for successful translational studies. All of this creates a virtuous cycle for improving computational models to the point at which they can drive compound selection for most DDD end points. When combined with more accurate in vitro testing, this may reduce and eventually eliminate animal test requirements (as recently indicated by FDA)145. A large number of trials are being conducted to identify binding modes of ligands and selection of the most energetically favored poses.
Aurigene Pharmaceutical Services Ltd. introduces Aurigene.AI™, an artificial intelligence (AI) and machine learning ... - Yahoo Finance
Aurigene Pharmaceutical Services Ltd. introduces Aurigene.AI™, an artificial intelligence (AI) and machine learning ....
Posted: Wed, 03 Apr 2024 07:00:00 GMT [source]
Outlook towards computer-driven drug discovery
The successful application of CADD approaches for the treatment of neurodegenerative disorders is also included in this review. Following are the steps required to perform a standard MD simulation (see Note 2 for additional MD techniques). Dr. Scott R. Lokey is a Professor of Chemistry in the Department of Chemistry and Biochemistry at the University of California, Santa Cruz. His research group focuses on the relationship between molecular structure and drug-like properties, especially cell permeability. Professor Lokey is also the director of the UCSC Chemical Screening Center, a high-throughput screening facility dedicated to early stage lead discovery, and is co-founder of Circle Pharma, a biotech startup focusing on the discovery of cyclic peptide inhibitors against intracellular targets. Unfortunately, CADD suffers from cognitive dissonance (bias to seek consonance) and also sloppy research that results from the lack of proper training.
We believe this review will be helpful for better understanding of CADD and its applications towards the discovery of new drug candidates against various fatal NDs. For larger system, more advanced MD techniques can be employed to enhance the sampling efficiency such as replica exchange methods. The protocols developed in our lab such as Hamiltonian replica exchange with biasing potentials (107) and replica exchange with concurrent solute scaling and Hamiltonian biasing in one dimension (108) are efficient replica exchange methods for use to enhance the MD efficiency. However, with all MD based methods the user must perform careful analysis to assure that the conformational ensemble is adequately converged for effective use in CADD. 1Conformational flexibility of molecules is a very important feature no matter if it is a small ligand or a large protein. Thus conformational sampling of a protein or ligand that produces an ensemble of biological meaningful conformations is necessary either for SBDD or for LBDD.
Although allowing comprehensive space coverage, the reaction path and success rate of generated compounds are unknown, and thus require computational prediction of their practical synthesizability. More recently, virtual on-demand chemical databases (fully enumerated) and spaces (not enumerated) allow fast parallel synthesis from available building blocks, using validated or optimized protocols, with synthetic success of more than 80% and delivery in 2–3 weeks (see the figure, part b). The virtual chemical spaces assure high chemical novelty and allow fast polynomial growth with the addition of new synthons and reaction scaffolds, including 4+ component reactions.
These are important metrics for the practical sustainability of on-demand synthesis because reduced success rates or unreasonable time and cost would diminish its advantage over custom synthesis. CADD methods are mathematical tools to manipulate and quantify the properties of potential drug candidates as implemented in a number of programs. These include a range of publicly and commercially available software packages; the subset described below represents examples of fundamental tools for CADD with emphasis on those commonly used in our laboratory. Al., the blue components are a part of computer-aided drug design (CADD) and it has transformed the way targets are being discovered and validated.
The process starts with the biological identification of a putative target to which ligand binding should lead to antimicrobial activity. In SDBB, the 3D structure of the target can be identified by X-ray crystallography or NMR or using homology modeling. LBDD is used in the absence of the target 3D structure with the central theme being the development of an SAR from which information on modification of the lead compound to improve activity can be obtained.
No comments:
Post a Comment